SURVIVAL ANALYSIS
Survival Analysis is a collection of methods designed for modeling time to an event of specific type. The event can be death, bankruptcy, hurricane, outbreak of mass protests or failure of a mechanical system. It can also be something good, like invention of a new drug. "Survival" up to a certain time means that the event has not occurred by that time.
In mainstream survival analysis the event in question may happen only once and is not subdivided into different categories. An example would be revocation of a medical license for controversial, borderline practices. In the recurring events framework the event may happen multiple times (e.g. engine failure). In the competing risks framework the event may be of multiple types. Each type may happen with a different likelihood and may require different treatment (e.g. death from different causes). Important research questions are the following.
- If
is the time until the first event or the time between two recurring events, estimate the survival curve, defined as
- How is the survival curve related to different characteristics of the system? For example, if we are modeling car accidents in a certain county, does the rate of accidents depend on the time of day? Is the rate different between male and female drivers? Alternatively, if we are modeling the time to the next default of a financial services company, how does the default rate vary with the overall state of the economy (say GDP) and debt-to-equity ratio of a randomly sampled company?
- If recurring events are the focus, can we see any pattern? Is the time between two subsequent events independent of what happened before? Or does the system learn from the past and the new survival curve incorporates information on previous occurrences? Are there valid contexts for renewal theory, which studies systems that reset themselves periodically?
- If there are many competing risks, are they correlated? Which one is likeliest at any specific time?
- What can we say about the survival of the whole system based on the survival of its components?
The major technical issue in survival analysis is censored data. This is best explained using an example. Assume we are estimating the survival profile of people who have just undergone certain treatment. Originally, there are n people in the experiment and during its course some of them die. However this does not happen to everybody. Some of the participants survive till the end of the experiment, while some others drop out of the experiment and lose touch. In each such case we never learn the complete lifetime. We only know that it is larger than the time of the last follow-up. So the right tail of the lifetime distribution is not observed or, as they say, the data are censored on the right. Most estimators in survival analysis are built to handle such censoring situations.
The estimation methods for survival curves can be split into two categories: nonparametric and parametric. The nonparametric methods are those which do not make any assumptions about the functional form of the survival curve. The most popular member of this class is the Kaplan-Meier estimator. Its calculation is quite simple. For expositional purposes let us refer to the event of interest as "death" from now on. Suppose there are
recorded times of death or censoring in the experiment, and they are ordered as
Let the number at risk of dying at time
be denoted
and the observed number of deaths be denoted
Then the Kaplan-Meier survival curve is given by
One can see that the Kaplan-Meier estimate is piece-wise constant, while the true survival curve may be somewhat smoother. Nonetheless, it has been shown that as the sample size converges to infinity the Kaplan-Meier estimate converges to the true survival curve. The plot below illustrates the Kaplan-Meier estimates run in an experiment containing two types of patients: those who received treatment and those who did not.
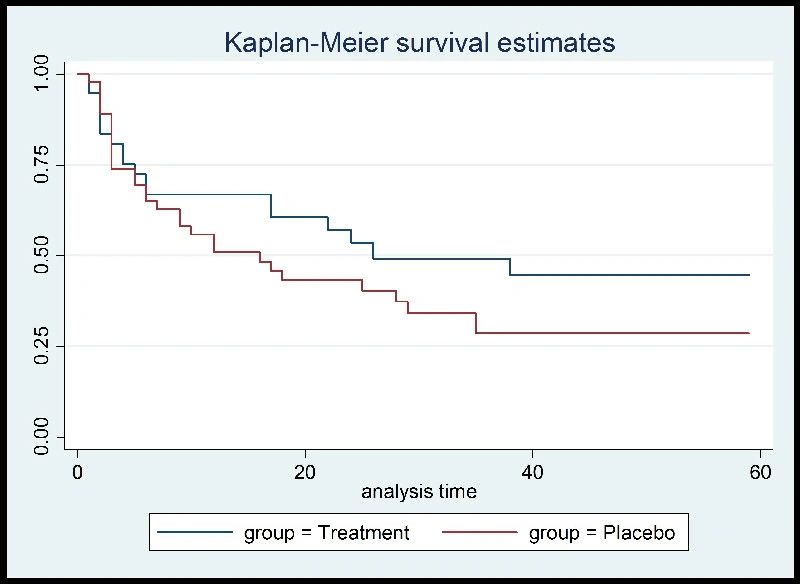
A log-rank test can be used to test whether the survival curves in two or more groups are the same. This is convenient when we need to study the effect of a single categorical predictor on the survival, with each category represented by a sufficient number of observations. For example, we might be interested in whether Caucasian, African American and Asian students complete an exam in the same amount of time (with not completing it all qualifying as a censored event). The log-rank test would be an appropriate tool for this problem. But what if we wanted to explore variability of the exam duration over many different characteristics of a student, some of them being continuous, like income in the family? We would not be able to represent all the distinct situations as different levels of a single categorical factor. We would need to build a multi-factor model capable of handling continuous predictors as well. This is where parametric methods come to use.
In parametric survival analysis we assume that the survival curve has a certain functional form, i.e. it is a known function of
some predictors (potentially) and some parameters. In many cases this implies that time to death belongs to one of the standard distributional families, e.g. exponential, Weibull, generalized gamma, lognormal, etc. In some other cases the functional form of the survival curve is complex enough to allow for richer risk profile. Still, most of the time we assume that the survival curve is absolutely continuous and, therefore, it can be written as
Here
is called the hazard rate (or death intensity, depending on the book). The hazard rate must be a non-negative function. Its meaning is that of actual death intensity: in a small time interval
the likelihood of death is approximately
The introduced notation means that, conditional on all the information available at time 0, the death at any future time follows a non-homegeneous Poisson process with intensity
Cox proportional hazards model links the survival probabilities to various predictors available at time 0. It attempts to capture effects similar to the following: women live longer than men, people with high income live longer than people with low income, cars with higher mileage break earlier, during off-peak periods a customer service specialist will be available more quickly, etc. A unit increase in each predictor is assumed to change the whole hazard rate by a certain factor. This is achieved by the following formula:
where
are predictors,
are coefficients and
is the baseline hazard rate, which can be any non-negative function of time. The proportionality assumption makes sense only in a subset of situations. However, as Cox has shown, it allows one to estimate
and
completely separately. Coefficients
are estimated using a version of partial maximum likelihood method, while
is estimated nonparametrically or parametrically. In stratified Cox model the baseline hazard rate is allowed to be different in different strata but the coefficients are the same.
In accelerated failure time model, not only does a change in a predictor shift the whole hazard rate curve, it makes time run faster (or slower):
where
Term
is sometimes referred to as the acceleration factor. If it equals 10, time runs 10 times as fast. Unlike Cox's framework,
is assumed to have known functional form, and so it can be estimated parametrically, via maximum likelihood, for example.
SURVIVAL ANALYSIS SUBCATEGORIES
SURVIVAL ANALYSIS REFERENCES
Lee, E. T., & Wang, J. W. (2003). Statistical Methods for Survival Data Analysis (3rd ed). Wiley-Interscience, Hoboken, New Jersey.
Balakrishnan, N., & Rao, C. R. (2004). Advances in Survival Analysis. Handbook of Statistics, Vol. 23. North Holland.
Cleves, M., Gould, W., & Marchenko, Y. (2016). An Introduction to Survival Analysis Using Stata (4th ed). Stata Press, College Station, Texas.
Kalbfleisch, J. D., & Prentice, R. L. (2002). The Statistical Analysis of Failure Time Data (2nd ed). Wiley-Interscience, Hoboken, New Jersey.
Rausand, M. & Høyland, A. (2004). System Reliability Theory: Models, Statistical Methods, and Applications. Wiley-Interscience, Hoboken, New Jersey. - This reference complements the traditional survival analysis literature because much attention is spent on the non-linear mechanism in which the survival of the whole system depends on the survival of separate components.
BACK TO THE STATISTICAL ANALYSES DIRECTORY
IMPORTANT LINKS ON THIS SITE